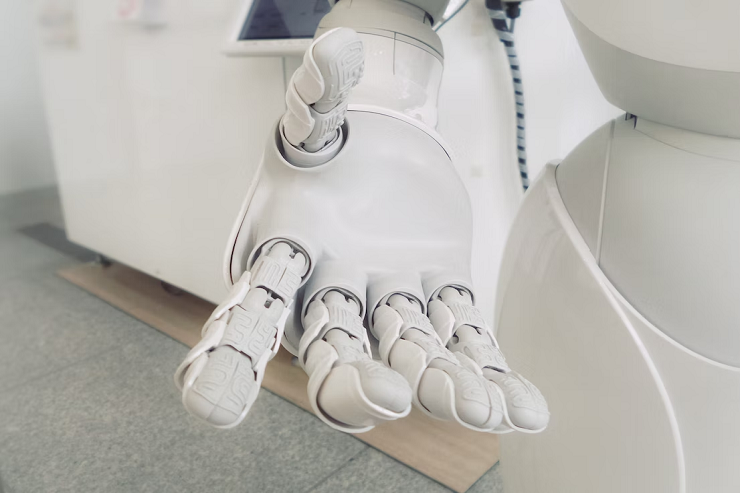
Welcome to the era of generative AI! With every passing day, artificial intelligence is becoming more and more advanced, and its application in various fields has become inevitable. One such field where AI is making a significant impact is data generation. Generative AI has revolutionized how we generate data by creating synthetic samples that look like humans produced them. And now, with active learning techniques, the future of generative AI looks even brighter!
In this blog post, we will explore how active Learning can take your generative AI models to new heights and show you how to use them effectively. So buckle up and prepare for an exciting ride into Active Learning in Generative AI!
What Is Generative AI?
Generative artificial intelligence (AI) is a subfield focused on generating new data from existing data. Generative AI can generate new images, videos, text, or data types.
There are two main types of generative AI: unsupervised Learning and reinforcement learning. Unsupervised Learning is a type of machine learning where the data is not labeled, and the algorithm has to learn from the data itself. Reinforcement learning is a type of machine learning where the algorithm is given feedback on its performance so it can learn to improve its performance over time.
Generative AI has many applications, including computer vision, natural language processing, and predictive modeling. It can be used to create realistic synthetic data for training machine learning models, to improve the accuracy of those models. Generative AI can also generate new products or ideas or find new patterns in data.
What Is Active Learning?
Active Learning is a type of machine learning that involves making predictions based on new data. The projections are made by using a model that has been trained on previously seen data. Active Learning is often used when there is a limited amount of training data available. It can also be used to improve the accuracy of the predictions made by the model.
Active Learning can be used for various tasks, including classification, regression, and clustering. Each study aims to learn a function that can map from input data to output labels. The procedure is known by training a model on a dataset. The model is then used to make predictions on new data.
- Classification is predicting whether an input belongs to one class or another. For example, you could classify images as a dog or a cat. To do this, you would train a model on a dataset of images labeled as containing either a dog or a cat. The model would learn how to discriminate between the two classes based on the features in the images. Once the model has been trained, it can predict new images.
- Regression is the task of predicting a constant value associated with an input. For example, expect the price of a house based on its size and location. You would train a model on a dataset of places labeled with their prices to do this. The model would learn how to predict the price of a house based on its size and location. Once the model has been trained, it can be used to predict new places.
- Clustering is the task of grouping inputs into clusters based on their similarity. For example, you could group customers into collections based on their purchasing habits. To do this, you would train a model on a dataset of customers labeled with their purchasing habits. The model would learn how to group similar customers into the same cluster. Once the model has been trained, it can predict new customers.
Active Learning is an effective way to make predictions when limited training data is available. It can also improve the accuracy of the model’s predictions by considering new data as it becomes available.
How Can Active Learning Be Used With Generative AI?
Active Learning is a powerful technique that can be used to train generative AI models. By using Active Learning, data scientists can select the most informative data points to label, which can significantly improve the performance of the AI model.
Active Learning is particularly well suited for generative AI models, as it can help reduce the amount of labeled data required to train the model. In addition, Active Learning can help to improve the accuracy of the generative AI model by selecting data points that are more likely to contain relevant information.
Active Learning can also reduce the time and resources required to train generative AI models, as the data scientist only has to label a small subset of the data. This allows developers to focus on fine-tuning the model rather than labeling large amounts of data.
What Are The Benefits Of Using Active Learning With Generative AI?
There are many benefits to using Active Learning with generative AI.
- For one, Active Learning can improve the accuracy of your generative AI models by allowing you to select the most relevant data for training.
- Additionally, Active Learning can reduce the data needed for training, speeding up the training process and saving on resources.
- Finally, Active Learning can also help improve the interpretability of your generative AI models by helping you understand which inputs are most important for generating the outputs.
How To Get Started With Active Learning And Generative AI?
Active Learning is a neural network pattern recognition technique and machine-learning methodology employed to use the data and eliminate bias effectively. It is a data-driven approach initiated by the user, who can be more selective in the use of data, which leads to improved results.
There are three primary types of active Learning:
- Semi-supervised: where the neural network is provided with a small amount of labeled data and must learn from this to generalize to the rest of the dataset;
- Unsupervised: where the neural network is only given input data and must learn from this without any external guidance;
- Reinforcement: where the neural network interacts with its environment, receiving feedback that will help it improve its performance over time.
The future of generative AI is Active Learning because it allows for better selection of data, improved results, and less bias.
- To get started with Active Learning, it is essential to have a good understanding of machine learning concepts such as supervised and unsupervised Learning, neural networks, and reinforcement learning.
- Additionally, having access to powerful computers and large data sets is essential for successful active Learning. Further, leveraging frameworks like TensorFlow or PyTorch can help you quickly build a dynamic learning model.
- Finally, it is necessary to understand the different types of AI models available and which one may best suit your application.
Once you understand active Learning, generative AI can be used to create new data from existing data, such as generating new images from existing images. Generative AI models use unsupervised Learning, which means they learn from unlabeled data and can create high-quality results.
- To get started with generative AI, it is essential to understand how neural networks work and the types of models available.
- Additionally, having access to powerful computers and large datasets is critical for successful generative AI.
- Finally, leveraging frameworks like TensorFlow or PyTorch can help you quickly build a generative AI model.
Conclusion
Active Learning is the next step in Generative AI’s evolution, and it can open up a wealth of possibilities for businesses and organizations. We hope this article has given you an understanding of what Active Learning is, how to use it, and why it will be so influential in the future of data-driven decision-making. As we continue to explore all that generative AI has to offer, we will discover even more ways that Active Learning can help us unlock more significant potential from our data sets.