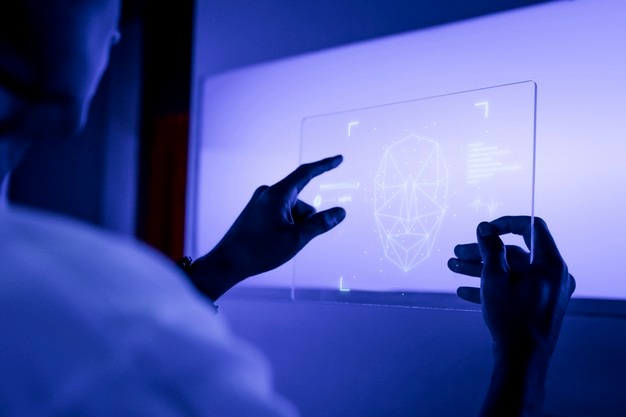
We are here to discuss the applications of machine learning (ML) in cyber security. However, before heading to (ML) and cyber attacks, one needs to understand the role of machine learning in computer science as a whole. ML is basically a branch of artificial intelligence that uses data and algorithms to emulate the way a human grasps and steadily improves its quality and accuracy.
With the use of statistical methods, algorithms are trained to classify or predict, unfold key insights in data mining projects. It can be helpful in decision-making for businesses and applications.
Role Of Machine Learning In Cyber Security
To understand the cyber attacks and develop a defensive response, ML can be helpful as it enhances the security process and makes it easy for IT security analysts to instantly recognize and deal with existing as well as new attacks.
1. Tasks Automating
With machine learning, one can develop a system, where repetitive and time-consuming tasks can be automated. For instance, malware analysis, network log analysis, triaging intelligence, and vulnerability assessments. With alone human effort, cyber security is not possible. Moreover, with the automation of tasks, cyber threats and attacks can be detected easily and remediated quickly.
Also, the automation feature can help reduce costs as the clients can do the upscaling and downscaling on their own without changing the employees’ requirements. This process of automation is referred to as AutoML.
2. Threat Detection And Classification
Algorithms of machine learning are utilised by applications to identify and respond to attacks. This could be achieved by reviewing big data(s) of security events and analysing the existing patterns of malicious attacks. When similar events happen, ML will be able to deal with the situation according to the requirement.
The dataset to support a machine learning model can be made by using Indicators of Compromise(IOCs). These can be helpful in real-time to monitor, identify and respond to threats. To define the malware behaviour, one can use the ML algorithms with IOC data sets.
At the time of the WannaCry ransomware crisis, Darktrace (a machine learning Enterprise Immune Solution, claims that they prevented the cyber attacks. David Palmer, Director of Darktrace technolgy, says, “Our algorithms spotted the attack within seconds in one NHS agency’s network, and the threat was mitigated without causing any damage to that organization,”
3. Phishing
Conventional phishing detection methods lack the accuracy and the speed to identify and differentiate between malicious and harmless URLs. Modern machine learning algorithm predictive URL classification models can detect the patterns that show malicious emails. Models are trained to check email headers, punctuation patterns, body copy and many more factors by which one can identify phishing attacks.
Multiple machine learning tools help to detect phishing emails via existing malicious emails patterns.
4. WebShell
WebShell is a code that is loaded into a website with malicious intentions to give access to the webroot directory of the network to make alterations. It allows hackers to gain database access which may include the personal information of the website users.
With the help of machine learning, the computer can detect whether the cart behaviour is general or malicious. Similarly, one can analyse the User Behaviour Analytics (UBA) that creates a supplementary layer above standard security measures to offer complete visibility, identify account compromises, and discover malicious insider activity. If something unusual happens such as the high number of downloads, late-night employee logins, etc. The user will get the risk score on the basis of activity, time and patterns.
5. Network Risk Scoring
Machine learning assesses cyberattack data and checks which networks areas were involved in specific attacks. Risk scores are given to network sections by using quantitative measures to aid corporates in prioritising resources. The obtained score is helpful to determine the probability and effect of the attack in a given network area. Therefore, machine learning proves to be a helping technology that declines the risk of being attacked again in the future.
While doing business profiling, you need to figure out which business areas are crucial; if attacked, can demolish the entire business. As per various businesses, these sections differs. For some, Customer Relationship Management (CRM) would be crucial or for others, the sales data could be vital.
It is imperative to understand the crucial sections of the business to give them more security and protect them from malware and other malicious attacks. Therefore, you must do business profiling and check the security arrangements frequently as the business and its nature keep changing, so its security measures should complement them too.
Conclusion
No matter, whether you are a big corporate or a small business, the cyber security threats are the same for all of you. In fact, small scale businesses are more prone to be victimised by cyber-attacks. You should involve machine learning in your cyber security arrangements to reduce the risk of attacks. Machine learning can read the existing attack patterns and assist the company in detecting malicious behaviour in various ways, as explained above. To know more about machine learning and cyber attacks, keep following our blog website. We post updates to prevent cyberattacks frequently.