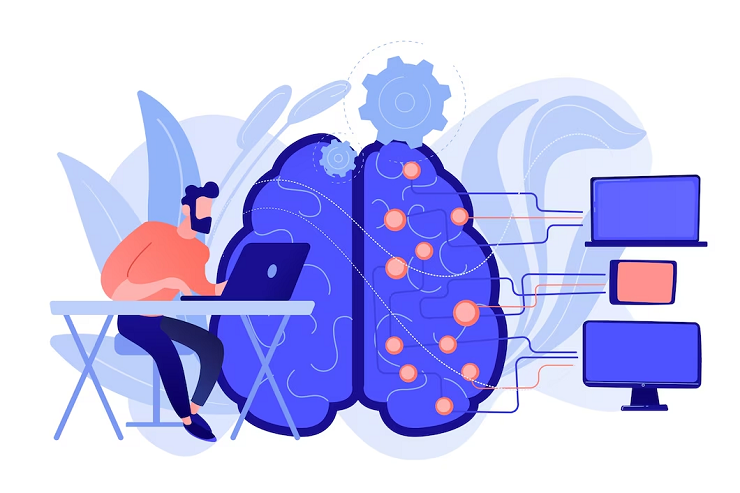
The rise of the internet has revolutionized how we live and work, but it has also brought new security challenges. Cyber attacks have become increasingly common, with hackers using sophisticated methods to access sensitive data and compromise systems. However, the development of machine learning technology has provided a powerful tool for predicting and preventing these attacks. In this blog post, we will explore the role of machine learning in cybersecurity and its potential to safeguard against future threats.
What Is Machine Learning?
Machine learning is a branch of artificial intelligence that allows systems to learn and improve from experience without being explicitly programmed automatically. It involves using algorithms that can analyze and identify patterns in data, which can then be used to make predictions or decisions. Machine learning has many applications, from natural language processing to image recognition and cybersecurity.
How Is Machine Learning Used In Cybersecurity?
In cybersecurity, machine learning algorithms analyze vast amounts of data from various sources, such as network traffic, log files, and system behavior, to identify potential threats. This process is known as threat detection. It involves using machine learning models to analyze and identify patterns in data that may indicate a cyber attack is imminent or underway.
- One of the key benefits of machine learning in cybersecurity is its ability to learn and adapt to new threats. Traditional security systems are often rule-based, meaning they can only detect known threats based on pre-defined rules. Machine learning models, on the other hand, can learn and adapt to new threats by analyzing data and identifying patterns that may indicate a new type of attack.
For example, a machine learning model can be trained to identify network traffic patterns indicative of a distributed denial-of-service (DDoS) attack. Once trained, the model can analyze incoming network traffic in real-time and identify ways that match those seen in previous DDoS attacks, allowing security teams to take action before the attack causes damage.
- Another use case for machine learning in cybersecurity is vulnerability detection. Machine learning models can analyze software code and identify potential vulnerabilities hackers could exploit. By identifying these vulnerabilities before they are used, organizations can take steps to patch them and prevent cyber attacks.
- Finally, machine learning can also be used for user behavior analysis. By analyzing user behavior patterns, machine learning models can identify abnormal behavior that may indicate a user has been compromised. For example, if a user suddenly starts accessing files and systems they don’t usually access, this could be a sign that their account has been compromised. Machine learning models can identify this bizarre behavior and alert security teams to take action.
Challenges In Using Machine Learning In Cybersecurity
While machine learning has the potential to revolutionize cybersecurity, some challenges need to be addressed.
- One of the biggest challenges is data quality. Machine learning models rely on high-quality data to learn and make accurate predictions. If the data used to train the model is of poor quality, the model will not be able to make accurate predictions, potentially leading to false positives or negatives.
- Another challenge is the need for expert knowledge to design and train machine learning models properly. Machine learning is a complex field requiring statistics, mathematics, and computer science expertise. Organizations need help finding and hiring experts with the necessary skills to design and train machine-learning models for cybersecurity.
- Finally, machine learning models can be susceptible to adversarial attacks. Adversarial attacks involve intentionally manipulating data in a way that causes the model to make incorrect predictions. For example, an attacker could modify network traffic to evade detection by a machine-learning model. This highlights the importance of continually training and updating machine learning models to ensure they are robust and can withstand adversarial attacks.
Conclusion
In conclusion, machine learning has the potential to revolutionize cybersecurity by providing powerful tools for predicting and preventing cyber attacks. By analyzing large amounts of data, machine learning algorithms can identify patterns indicating an attack is imminent or underway, allowing security teams to take action before significant damage occurs. However, some challenges need to be addressed, such as data quality, the need for expert knowledge, and the potential for adversarial attacks.
To address these challenges, organizations must invest in high-quality data and hire experts with the necessary skills to design and train machine learning models for cybersecurity. They must continually update and refine these models to ensure they are robust and can withstand adversarial attacks.
As the threat landscape evolves, machine learning will become an increasingly important tool in the fight against cyber attacks. By leveraging the power of machine learning, organizations can stay one step ahead of hackers and protect their valuable assets from harm.